Craig receives NIH grant to improve radiologists' breast cancer screening performance
The work will focus on how visual adaptation may facilitate the reading of breast cancer screening image batches.
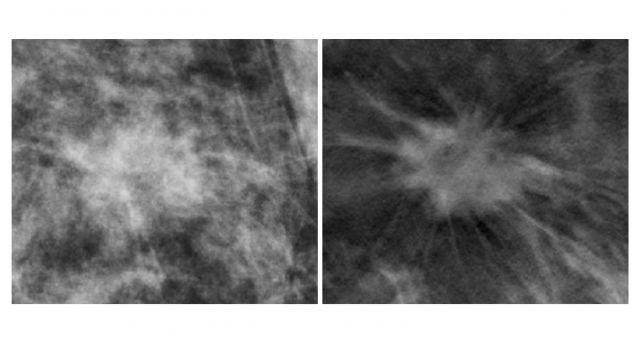
VIU Lab member Craig Abbey is one of four principle investigators of a newly awarded National Institutes of Health (NIH) grant, entitled Sequential Reading Effects in Digital Breast Tomosynthesis, with funding provided by the National Cancer Institute (NCI). The grant investigates visual adaptation as a mechanism for improved performance in sequential batch reading in breast cancer screening images (Figure 1).
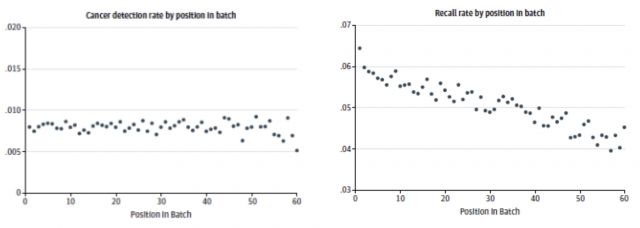
Radiologists often read high-volume exams, such as breast-cancer screening images, in uninterrupted batches. Historically there has been concern that this practice would lower accuracy due to decreased vigilance. However, a number of studies in the US and Europe have found the opposite effect (Figure 2), with batch reading leading to higher accuracies (Burnside et al., 2005) that continue to improve as readers progress through an image batch (Taylor-Philips et al., 2017; Abbey et al., 2020). Currently, there is no accepted explanation for this improvement.
This grant will explore visual-cognitive adaptation as a mechanism for this improvement. Visual-cognitive pathways are able to recalibrate in response to new visual environments, and we hypothesize that this process leads to the observed improvements in batch reading diagnostic accuracy. If confirmed, this explanation could have important implications for how to improve and optimize radiologist performance in breast cancer screening.