Two new short papers at the International Conference on Learning Representations (ICLR 2021) comparing human and AI capabilities to infer direction of gaze and understanding of scenes
The work mutually improves our understanding of how the computational processes mediating artificial intelligence (AI) compare to those employed by humans for common and important real-world tasks
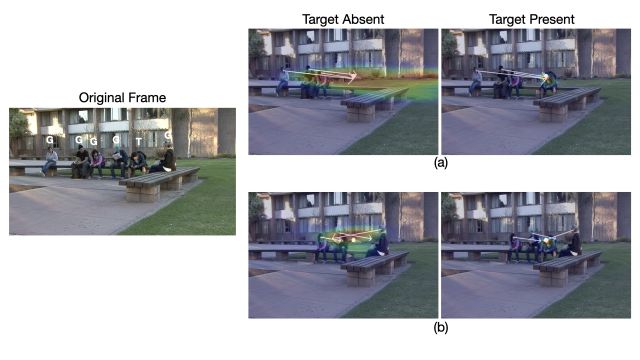
The first VIU Lab talk of the Ninth International Conference on Learning Representations (ICLR 2021 virtual conference) was delivered by VIU PhD student Nicole Han. Nicole presented her work on the similarities and differences between humans and a state-of-the-art CNN-based deep learning model estimating where another person is looking based on an image of their face (i.e., gaze perception). Nicole and her co-authors found a significant correlation between the decisions that humans and artificial intelligence agents (AI) make about a particular person's gaze direction from real-world images of multiple individuals all looking at one "target" person. Crucially, the AI and the human subjects arrived at their similar inferences through fundamentally distinct information processes. Human inferences were sensitive to "scene context" information, such as the number of people in the scene, the presence of the target person, etc. The AI's decisions, on the other hand, were largely insensitive to this higher-order relational information, indicating either a failure to learn these relationships or a computational feature that intentionally discounts this information. Nicole's talk can be seen in full here on her YouTube channel.
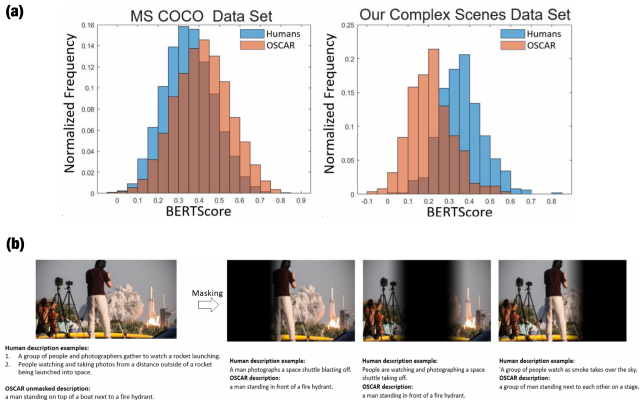
The second VIU Lab paper at ICLR 2021 was presented by PhD student Shravan Murlidaran on how humans and AI infer meaning and context from the complex scenes we encounter everyday; that is, how machines and humans represent an "understanding" of the scene. Recent advances in natural language processing and computer vision have led to AI models that interpret simple scenes at human levels. Yet, a complete understanding of how humans and AI models differ in their interpretation of more complex scenes has remained elusive. Shravan and colleagues created a dataset of complex scenes depicting myriad typical human behaviors and social interactions. Humans and AI agents described each scene with a sentence (captioning), and these descriptions were evaluated according to their similarity to “ground truth” descriptions that were acquired separately from five other humans. The results show that increasing scene complexity greatly reduces the similarity between the ground truth and AI descriptions while similarity between different humans' descriptions remained high. Further, the authors showed that occluding different regions of the images changed the human and AI scene descriptions in different ways, suggesting that humans and AI rely on different objects/regions for scene understanding. Finally, human descriptions for some of the images were more sensitive to the presence of any occluder (i.e., across occlusion positions) than those of the AI, suggesting that humans integrate information across the entire spatial layout of the image for scene understanding. Together, the results are a first step toward understanding how machines fall short of human visual reasoning with complex scenes depicting human behaviors. A pre-print of Shravan’s paper can be seen in full here on arXiv.