New NAACL 2022 paper by Yujie Lu improves AI NLP through "Imagination-Augmentation"
The Imagination-Augmented Cross-modal Encoder (iACE) incorporates external knowledge from generative networks and pre-trained vision-and-language models
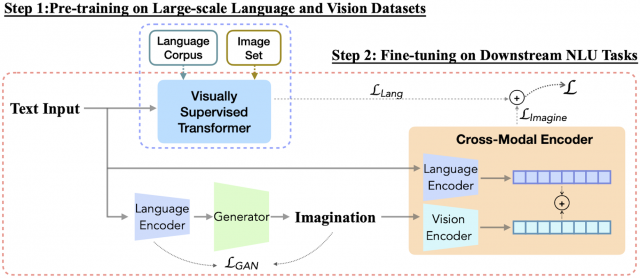
Human brains integrate linguistic and perceptual information simultaneously to understand natural language, and hold the critical ability to render imaginations. Such abilities enable us to construct new abstract concepts or concrete objects, and are essential in involving practical knowledge to solve problems in low-resource scenarios. However, most existing methods for Natural Language Understanding (NLU) are mainly focused on textual signals; they do not simulate human visual imagination ability, which hinders models from inferring and learning efficiently from limited data samples.
In "Imagination-Augmented Natural Language Understanding", a new paper accepted for presentation at the NAACL 2022 Main Conference, a team led by first author and VIU graduate student Yujie Lu introduce an Imagination-Augmented Cross-modal Encoder (iACE) to solve natural language understanding tasks from a novel learning perspective -- imagination-augmented cross-modal understanding. iACE enables visual imagination with external knowledge transferred from the powerful generative and pre-trained vision-and-language models. Extensive experiments on GLUE and SWAG show that iACE achieves consistent improvement over visually-supervised pre-trained models. More importantly, results in extreme and normal few-shot settings validate the effectiveness of iACE in low-resource natural language understanding circumstances.
Yujie's creative and novel work, done in collaboration with VIU PI Miguel Eckstein and Mind & Machine Intelligence Co-Director William Wang, can be explored in full here on arXiv.