Perceptual Learning
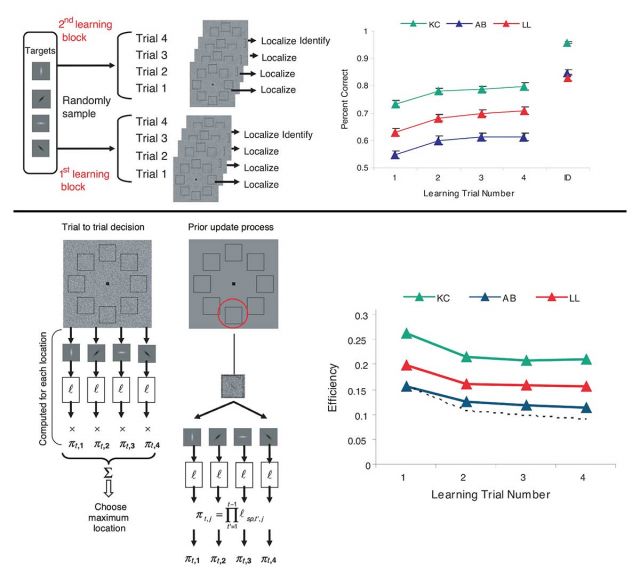
When people first engage new perceptual tasks, such as recognizing and/or searching for novel objects or faces, we are often uncertain about which visual properties we should use (i.e., task-relevant "target" features) and which we should ignore (i.e., task-irrelevant "distractor" features; Eleanor Gibson, 1969, 1991). With practice, humans improve their performance by learning, either through explicit training of feature-relevance or implicit associations gained through performance feedback, to use features that are relevant to the task while ignoring those that are irrelevant ones. We have developed a new paradigm (optimal learning paradigm, OPL) that allows to compare how well humans learn (via attentional weighting optimization) compared to an optimal Bayesian learner. This approach allows to us to identify the sources of sub-optimality in human perceptual learning for specific tasks, understand why the brain's architecture may lead to such sub-optimal behavior, and guide the creation of programs for improving learning.