Computational Tools & Models
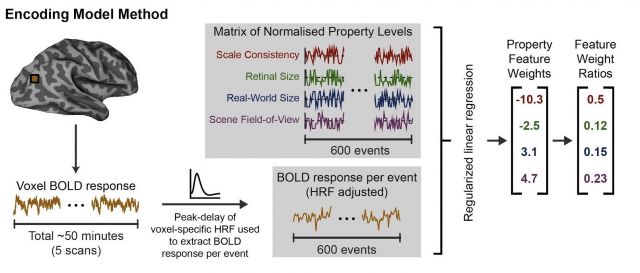
Since its inception, the VIU Lab has focused on the development of formal computational and statistical models for the principled, quantitative testing of hypothesized perceptual and cognitive mechanisms. Bayesian models derived from Signal Detection Theory have always comprised the core class of VIU modeling, with Bayesian Ideal Observer theory offering major contributions to many of our lab's papers. More recently, we have incorporated models from the fields of computer science and machine learning, with a special emphasis on deep learning methods (e.g., convolutional and recurrent neural networks). Analysis of neuroimaging (functional magnetic resonance imaging, fMRI) and electro-encephalography (EEG) data cull from a host of computational tools, including multivariate pattern classifiers, feature-encoding models, and representational similarity.
Ultimately, we seek coherent unified explanations for cognitive and perceptual phenomena by using computational modeling to instantiate formal, mechanistic, quantitative models of information processing. The grand goal is the discovery of explicit formal models that reliably predict our perceptual experience and decision making (psychophysics), our active information-seeking mechanisms (eye tracking), and the neural computations and representations that give rise to our experiences, beliefs, and actions (neuroimaging and electrophysiology).