Visual Search & Attention
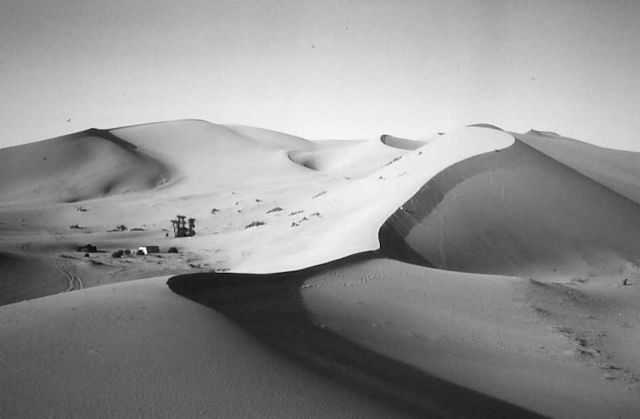
Whether looking for house keys, a friend in the crowd, or a car in the parking lot, visual search is one of our most important and ubiquitous real-world tasks. Beyond these common everyday examples, visual search plays a critical role in many high-stakes scenarios, both historically (e.g., ancient hunter-gatherers hunting and foraging for food) and in modern civilization (e.g., looking for tumors in a medical image, or potentially dangerous objects in a TSA X-ray inspection). Visual search involves moving our eyes (and the high-resolution fovea) and attention to examine different regions of interest within a scene. Our laboratory tries to understand the mechanisms that control how humans deploy attention and eye movements, and identify the strategies and neural computations the brain uses to optimize visual search.
Our premise is that computational modeling, when combined with eye tracking technology, allows us to rigorously define and test hypothesized neural computations that inform these deployment strategies, helping to bridge the many disciplines interested in search, attention and eye movements (e.g., psychology, cognitive neuroscience, neurophysiology). In addition, we study how humans actually look for things outside the lab by measuring natural behavior and eye movements while people perform real-world search tasks, such as radiologists looking for tumors in medical images and fishermen looking for schools of sardines and/or tuna on the ocean surface.
Our research has concentrated on using signal detection theory (especially Bayesian Ideal Observers) to both 1) Derive models that optimize the use of visual information for the guidance of search and formation of perceptual decisions (i.e.,how information should be used), and 2) Explain human inefficiencies by adding hypothesized mechanisms to these ideal models that are sub-optimal for a particular search task (i.e., how information actually is used by humans). We rigorously and quantitatively test the ability of these models to predict human performance and eye movements, and compare their ability to that of computational implementations of competing alternatives found in the literature, including limited resources, serial attention, and guided search models.
Affiliated Researchers